In the wake of 2015/2016 microservice hype, my tech-adjacent leadership struggled to understand “what a microservice is”, and whether they should push their organizations to transition to microservices (or allow their engineers to push them toward that end). Upon request, I gave this talk on microservices for non-technical audiences to distill tangible wisdom and add advice.
Tag: slides
I gave the keynote address at Byte Sized, a workshop for middle school girls spearheaded by SAIL ON students. My First AI (or: Decision Trees & Language Modeling for Middle Schoolers) solidified the basics of artificial intelligence and the if/else
statements taught the previous day.
The talk introduces the language identification problem within AI, teaches about decision trees, and then asks students to write decision trees in small groups to distinguish between Hmong, Balinese, Zulu, and other languages. After a debrief on why computers are might be more effective than human-written rules, it briefly ties in themes of feature extraction and gradient descent via GBMs.
As the progenitor and leader of SAIL ON, the Stanford AI Lab’s year-round effort to attract and keep underrepresented minorities in the field of Artificial Intelligence, I engage high schoolers about artificial intelligence, machine learning, and the positive social impacts of our field. SAIL ON meets once a month in the Computer Science building at Stanford. Its trifold focus allows past participants in the SAILORS two-week summer camp to continue to learn about AI, to nurture strong relationships with each other, and to lead outreach projects that bring the technical, humanistic, and diversity missions of the AI outreach program to the wider community.
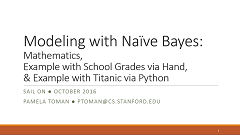
As the educational component of the October meeting of SAIL ON, we discussed and applied Naive Bayes modeling. Like other machine learning methods, Naive Bayes is a generic approach to learning from specific data such that we can make predictions in the future. Whether the application is predicting cancer/whether you’ll care about an email/who will win an election, we can use the mathematics of Naive Bayes to connect a set of input variables to a target output variable. (Of course, some problems are harder than others!)
We focused on the derivation of Naive Bayes with a chalk-and-talk discussion (slides), identifying why Naive Bayes is mathematically justified and posing some deeper thought questions. We checked understanding with a hand-calculation of a Naive Bayes problem (handout): does a shy student received an A in a class, given some observations about her and some observations about more forthcoming students? We then turned to a Jupyter Notebook that applies the same methods on a larger scale, working on the Titanic challenge from Kaggle with an applied introduction to pandas and sklearn: given passenger manifest records, can we predict who survived?
By providing this basis, I hope to increase appreciation for applications of what students are seeing in their math classes, and to facilitate students moving further on their own with applied machine learning before November’s meeting.
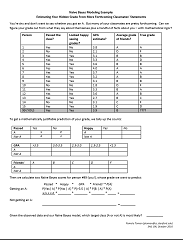
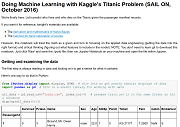
Convolutional neural networks transformed computer vision from “extremely hard” to “trivially achievable after a few weeks of coursework” between 2012 and 2016.
I prepared a talk for technical professional audiences that describes how neural networks extend linear classification, intuitions behind why convolutional neural networks work well for vision, and the circumstances in which they’re worth consideration. I used the “Intro to CNNs for Computer Vision” materials at two different employers in 2016, and also with the high schoolers who participated in SAIL ON in 2017. (SAIL ON extended a Stanford summer program in AI that captured underrepresented minorities; I led the summer program and extended it to two years of follow-up monthly outreach.)
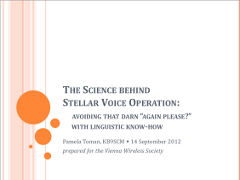
In noisy conditions on the airwaves, it can be hard to exchange information effectively. Rather than throwing more power or another $1000 of equipment at the problem, radio operators can often improve reception by adjusting the signal at its source: their articulatory organs. By enunciating, focusing on vowels, using recognized phonetic alphabets, and matching listeners’ expectations about pitch, amateur radio operators can effectively boost the quality of their signal.
To download the presentation in .pdf format, click on the image at right or the preceding link.
Context for Non-Hams
Relevance of Amateur Radio
Since the 1960s and 1970s, public interest in amateur radio has waned as reliable mobile communication has become available for minimal cost. Our dependence on such systems, however, has left us increasingly vulnerable to natural and man-made disasters. When the communication infrastructure is destroyed or severely overloaded (such as the 2011 Japanese earthquake and tsunami, Hurricane Katrina, the Marine Corps Marathon, and presidential inaugurations), hams continue to provide robust, decentralized communication.
Beyond practical uses, ham radio is also a hobby like any other, worthwhile for the enjoyment it brings.
“Noise” on the Air
The amateur radio bands do not always provide a perfect channel for communication. In especially bad conditions, trying to understand a message can be akin to listening to shouting from half a block away, on a windy day with city traffic. Although some atmospheric and noise conditions are uncontrollable, amateur radio operators do our best to produce cleanly intelligible signals, and we hone the skills required to understand content despite bad conditions.